THE CENTER OF GRAVITY FOR ENTREPRENEURS IN TEXAS

Austin Deep Learning
JC – Journal Club
Title:
# ENCODING WORD ORDER IN COMPLEX EMBEDDINGS
Authors:
Benyou Wang, Donghao Zhao, et al.
This paper will be presented by:
Connor Favreau
Abstract:
Sequential word order is important when processing text. Currently, neural networks (NNs) address this by modeling word position using position embeddings. The problem is that position embeddings capture the position of individual words, but not the ordered relationship (e.g., adjacency or precedence) between individual word positions. We present a novel and principled solution for modeling both the global absolute positions of words and their order relationships. Our solution generalizes word embeddings, previously defined as independent vectors, to continuous word functions over a variable (position). The benefit of continuous functions over variable positions is that word representations shift smoothly with increasing positions. Hence, word representations in different positions can correlate with each other in a continuous function. The general solution of these functions is extended to complex-valued domain due to richer representations. We extend CNN, RNN and Transformer NNs to complex-valued versions to incorporate our complex embedding (we make all code available). Experiments 1 on text classification, machine translation and language modeling show gains over both classical word embeddings and position-enriched word embeddings. To our knowledge, this is the first work in NLP to link imaginary numbers in complexvalued representations to concrete meanings (i.e., word order).
Paper:
https://arxiv.org/pdf/1912.12333.pdf
Spots are limited to keep the discussions organized.
Austin Deep Learning Journal Club is group for committed machine learning practitioners and researchers alike. The group meets every first Tuesdays of each month to discuss research publications. The publications are usually the ones that laid foundation to ML/DL or explore novel promising ideas and are selected by a vote. Participant are expected to read the publications to be able to contribute to discussion and learn from others. This is also a great opportunity to showcase your implementations to get feedback from other experts.
Anyone can suggest and vote for the next paper on Austin Deep Learning slack work space (#paper_group channel): https://austin-deep-learning-slack.herokuapp.com/
Please only RSVP if you are certain that you will be participating.
What to bring:
A copy of the paper (either digital or hardcopy)
By Appointment Only
Our doors are open! Reach out to Members@CapitalFactory.com to book your private, in-person membership tour.
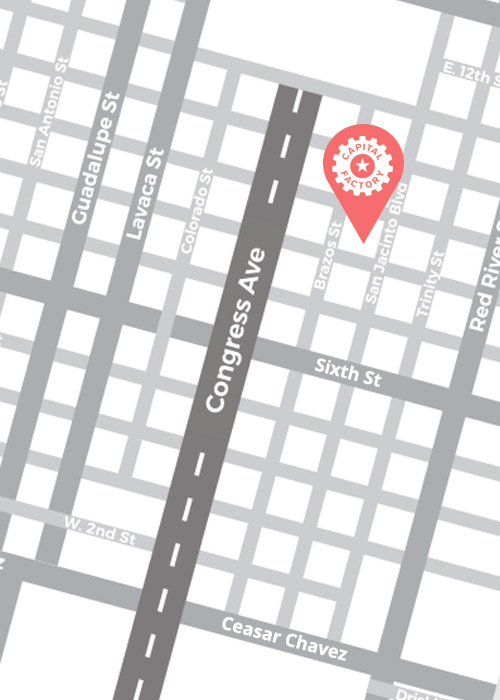